Precise Insights
Advanced Data and AI for Lung Cancer
On-demand access to the largest and most sophisticated oncology data for accelerated RWE and improved patient outcomes.
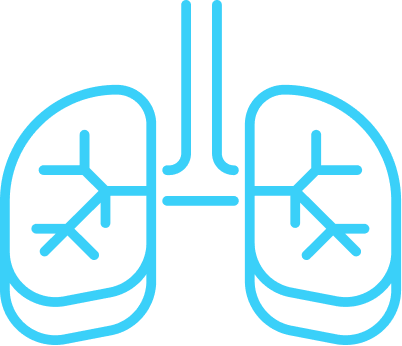
See some of the 1000s of data elements we offer:
labs
Results from variety of patient tests
Visits
Oncology practice visits
Observations
Tumor-related observations plus vitals, biometrics, pain, and more
Procedures
Surgeries, radiation, biopsies, imaging, chemotherapy, etc.
Medications
Start and end dates, brand vs. generic codes, dosage, duration, and cycles
Diagnoses
Disease state, status, severity, and metastasis
full ngs panel
Complete genomic report with treatment exposure and clinical outcomes
Disease Progression
Directly-observed measures of critical endpoints
Adverse Events
Different types of adverse responses
Histology
Classification into multiple categories
Cost & Utilization
Adjudicated costs with linked claims data
Safety, Comorbidities
Pre-cancer and claims charge events
Specialty Pharmacy & Hub
Rx acquisition status details
Payer & Formulary
Drug tiers and coverage
Social Determinants
Social and physical environment factors
NSCLC Histology Classifier
Segment histology into four categories
Cancer Subtype
Cancer type when ICD code is unknown
ECOG
Predict oft-missing performance score
Metastatic Status
Impute missing data from unstructured notes
Date of Initial Dx
Impute index event
EGFR Status
Impute specific gene mutation
Line of Therapy
Regimen or progression-based drug classes
Patient Adherence
Identify root cause of product switching
Patient Acquisition
Predict factors driving patients’ brand decision
Request Data Count
Explore ConcertAI datasets to see how many patients are in your disease area and meet study criteria.
lung cancer
Research Studies
Our scientists regularly publish leading RWE studies in the fields of clinical development and health economics and outcomes research.
lung cancer research study
Impute Subtype
Cancer subtypes are rarely captured in structured EMR data but are important for clinical outcomes and cost analyses. ConcertAI developed an AI model to identify NSCLC patients from a heterogeneous cohort of lung cancer patients.
See Full StudyMethods
Machine Learning Model
Analysis
- Treatment patterns
- Clinical effectiveness
- Progression-free survival
- Overall survival
Patient Features
- Lab Tests
- Staging Information
- Surgery Information
- Medications Administered
Results
56,748
Patients labeled as NSCLC or not-NSCLC based on expert nurse abstraction
This Data was divided into three sets:
Train
Test
Validate
The model improved accuracy:
Test machine learning model
Best-performing rules-based model
The model had strong relevance scores:
Precision
Recall
Conclusion
We can develop and validate reliable machine learning models to label lung cancer subtypes. This could save substantial time and effort compared to expert manual curation.
Research for ISPOR, 2020
lung cancer research study
Predict Slow Progressors
Few clinical characteristics of patient with exceptional response to existing cancer therapies are known. ConcertAI trained a deep learning model to predict slow progression, a proxy, in aNSCLC in the second line setting.
See Full StudyMethods
Machine Learning Model
Patient Criteria
- Pathologically confirmed aNSCLC without other primary cancer Dx
- Started 2nd-line therapy between 2013 and 2017
- Labeled slow progressors if no evidence of progression or death within 180 days of index
Results
2,205
Patients met selection criteria of the study
patients labeled slow progressors:
The model improved accuracy:
Test machine learning model
Best-performing logistic regression model
The model had strong relevance scores:
Precision
Recall
Conclusion
This deep learning model may be useful in discovering novel drivers of favorable response.
Research for ASCO, 2020